Table Of Content
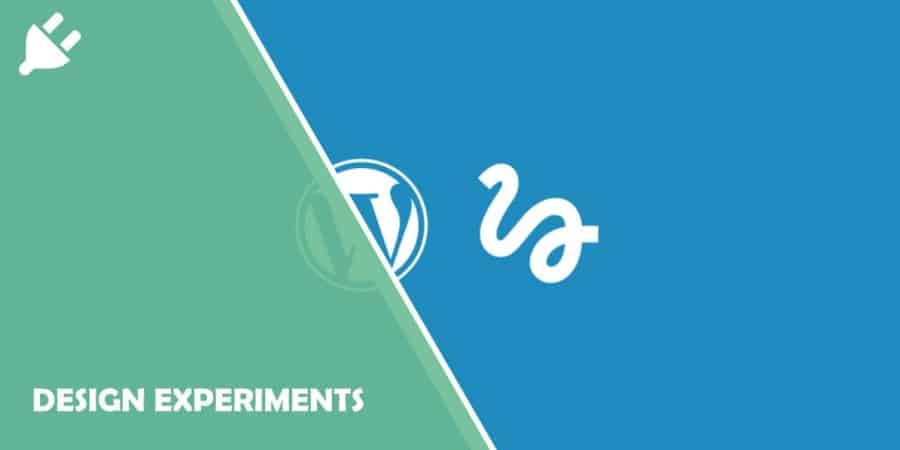
In this example we wish to determine whether 4 different tips (the treatment factor) produce different (mean) hardness readings on a Rockwell hardness tester. The treatment factor is the design of the tip for the machine that determines the hardness of metal. In the first example provided above, the sex of the patient would be a nuisance variable. For example, consider if the drug was a diet pill and the researchers wanted to test the effect of the diet pills on weight loss.
Statistical Analysis of the Latin Square Design
Here we have used nested terms for both of the block factors representing the fact that the levels of these factors are not the same in each of the replicates. We now illustrate the GLM analysis based on the missing data situation - one observation missing (Batch 4, pressure 2 data point removed). The least squares means as you can see (below) are slightly different, for pressure 8700.
Example 4.1: Hardness Testing
But what if that person has a different launch style than I do? Or what if the popsicle sticks and rubber band they use are so different from the catapult that I use that there’s a difference in how the gummi bears launch? Some launches will be different from other launches, so I still wouldn’t be collecting all of my data under stable conditions. The most typical reason for dealing with blocks is to handle variables that you cannot manipulate. The blocking and confounding techniques in 2k factorial design of experiment is described in Video 1.
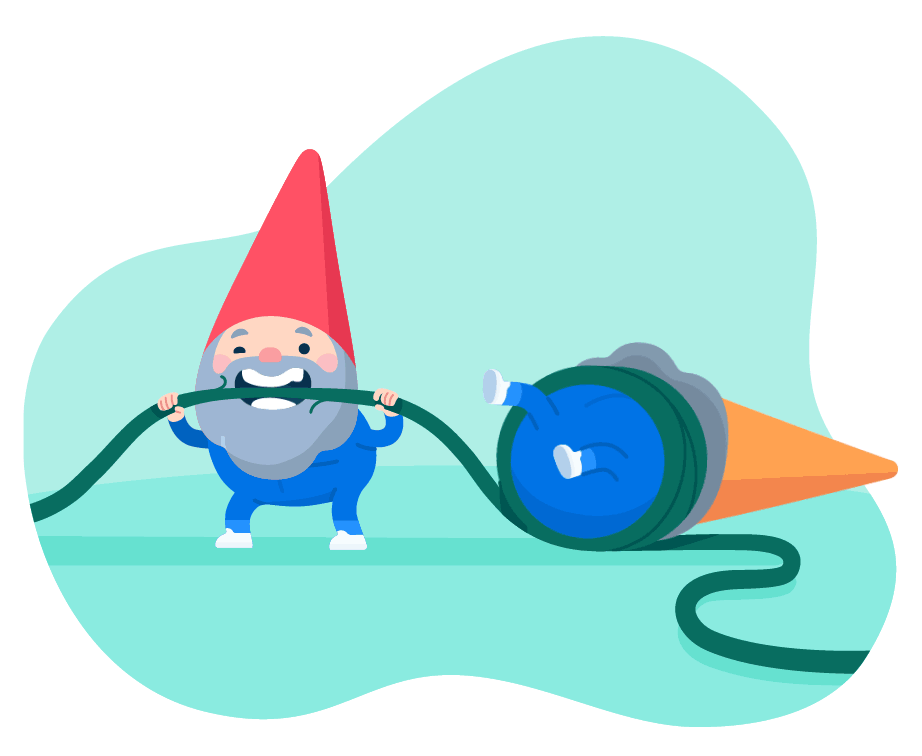
Comparing the CRD to the RCBD
The blocking factor is usually not a primary source of variability. An example of a blocking factor may include eye color of a patient, so if this variability source is controlled, greater precision is achieved. Completely randomized design is where the groups are chosen at random. In fact, completely randomized design has been considered the most efficient over the years. As the 2k design is primarily used to screen factors/variables, often a very large number of experimental units are required to complete even one full replication. For an example, 26 design with six variables requires 64 experimental units to complete one full replication.
Human ACE2 peptide-mimics block SARS-CoV-2 pulmonary cells infection Communications Biology - Nature.com
Human ACE2 peptide-mimics block SARS-CoV-2 pulmonary cells infection Communications Biology.
Posted: Fri, 12 Feb 2021 08:00:00 GMT [source]
Note that the A effect and the B effect are orthogonal to the AB effect. This design gives you complete information on the A and the B main effects, but it totally confounds the AB interaction effect with the block effect. In this case treatment (1) and treatment ab will be in the first block, and treatment a and treatment b will be in the second block. Then randomly permute the order of the columns to yield the final square for the experimental layout. Where “i” is the index for replicates and “j” is the index for blocks within the replicates.
Whenever, you have more than one blocking factor a Latin square design will allow you to remove the variation for these two sources from the error variation. So, consider we had a plot of land, we might have blocked it in columns and rows, i.e. each row is a level of the row factor, and each column is a level of the column factor. We can remove the variation from our measured response in both directions if we consider both rows and columns as factors in our design. Randomized block design (RBD) takes advantage of grouping similar experimental units into blocks or replicates. One requirement of RBd is that the blocks of experimental units be as uniform as possible.
He pointed out that it would be unusual to use blocks in design of experiments as a way to check for the need for further experimentation. The reason is that, so far, I’ve been considering only variables that we can measure and manipulate. As the name implies, random experimental design involves randomly assigning experimental conditions.
Balanced Incomplete Block Design (BIBD)
The general linear test is the most powerful test for this type of situation with unbalanced data. Here we have four blocks and within each of these blocks is a random assignment of the tips within each block. The nuisance factor they are concerned with is "furnace run" since it is known that each furnace run differs from the last and impacts many process parameters. The conf.design package in R is described by its author as a small library contains a series of simple tools for constructing and manipulating confounded and fractional factorial designs. The function conf.design can be used to construct symmetric confounded factorial designs. I could, of course, have someone else launch bears at the same time I do, so that I could get more data faster.
Therefore, this is not a balanced incomplete block design (BIBD). Situations where you should use a Latin Square are where you have a single treatment factor and you have two blocking or nuisance factors to consider, which can have the same number of levels as the treatment factor. The degrees of freedom for error grows very rapidly when you replicate Latin squares. But usually if you are using a Latin Square then you are probably not worried too much about this error. The error is more dependent on the specific conditions that exist for performing the experiment.
Although remarkable advancements have been achieved in generative models, very few efforts have been paid to design relevant quality assessment models. In this paper, we propose a novel blind image quality assessment (IQA) network, named AMFF-Net, for AGIs. AMFF-Net evaluates AGI quality from three dimensions, i.e., "visual quality", "authenticity", and "consistency". After that, an Adaptive Feature Fusion (AFF) block is used to adaptively fuse the multi-scale features with learnable weights.
The simplest case is where you only have 2 treatments and you want to give each subject both treatments. Here as with all crossover designs we have to worry about carryover effects. In this factory you have four machines and four operators to conduct your experiment. Use the animation below to see how this example of a typical treatment schedule pans out. When the data are complete this analysis from GLM is correct and equivalent to the results from the two-way command in Minitab.
A non-blocked way to run this experiment would be to run each of the twelve experimental wafers, in random order, one per furnace run. That would increase the experimental error of each resistivity measurement by the run-to-run furnace variability and make it more difficult to study the effects of the different dosages. The blocked way to run this experiment, assuming you can convince manufacturing to let you put four experimental wafers in a furnace run, would be to put four wafers with different dosages in each of three furnace runs.
The only randomization would be choosing which of the three wafers with dosage 1 would go into furnace run 1, and similarly for the wafers with dosages 2, 3 and 4. The usual rule is to pick an effect you are least interested in, and this is usually the highest order interaction, as a means of specifying how to do blocking. In this case it is the AB effect that we will use to determine our blocks. As you can see in the table below we have used the high level of AB to denote Block 1, and the low-level of AB to denote Block 2. Blocking first, then randomizing ensures that the treatment and control group are balanced with regard to the variables blocked on.
What if the missing data point were from a very high measuring block? It would reduce the overall effect of that treatment, and the estimated treatment mean would be biased. Before high-speed computing, data imputation was often done because the ANOVA computations are more readily done using a balanced design. There are times where imputation is still helpful but in the case of a two-way or multiway ANOVA we generally will use the General Linear Model (GLM) and use the full and reduced model approach to do the appropriate test. Often in medical studies, the blocking factor used is the type of institution. Blocking is one of those concepts that can be difficult to grasp even if you have already been exposed to it once or twice.
No comments:
Post a Comment