Table Of Content
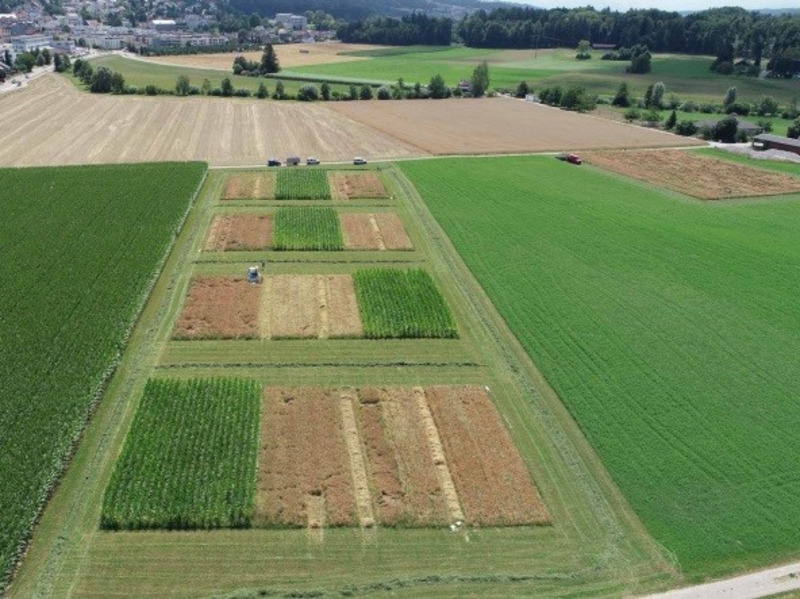
The RCBD utilizes an additive model – one in which there is no interaction between treatments and blocks. The error term in a randomized complete block model reflects how the treatment effect varies from one block to another. Implementing blocking in experimental design involves a series of steps to effectively control for extraneous variables and enhance the precision of treatment effect estimates.
Performance Analysis of Shunt Network for Adaptive Optical Hybrid Interconnection
In this case, the inference made on the significance of the factor can be extended to the whole population but the factor effects are treated as contributions to variance. When we have missing data, it affects the average of the remaining treatments in a row, i.e., when complete data does not exist for each row - this affects the means. When we have complete data the block effect and the column effects both drop out of the analysis since they are orthogonal. With missing data or IBDs that are not orthogonal, even BIBD where orthogonality does not exist, the analysis requires us to use GLM which codes the data like we did previously. Here we have two pairs occurring together 2 times and the other four pairs occurring together 0 times.
Blocking in experimental design
But I have other things to do besides launch gummi bears, so it's unlikely I can complete my experiment in one day. If I have to launch some bears on a day when it’s cold and rainy outside and some bears on a day when it’s sunny and mild, there might be an effect on how the gummi bears launch. BlockingWith small samples, it's possible to randomly divide the subjects and still get different groups. Randomly dividing the subjects into the 2 groups is the most likely to make the treatment and control groups as alike as possible because it eliminates human bias. Not only differences that the researcher has identified as relevant, but on all characteristics, including the hidden ones that the researcher might not realize are important.
What else is there about BIBD?
What we now realize is that two blocking factors is not enough! It looks like day of the week could affect the treatments and introduce bias into the treatment effects, since not all treatments occur on Monday. We want a design with 3 blocking factors; machine, operator, and day of the week.
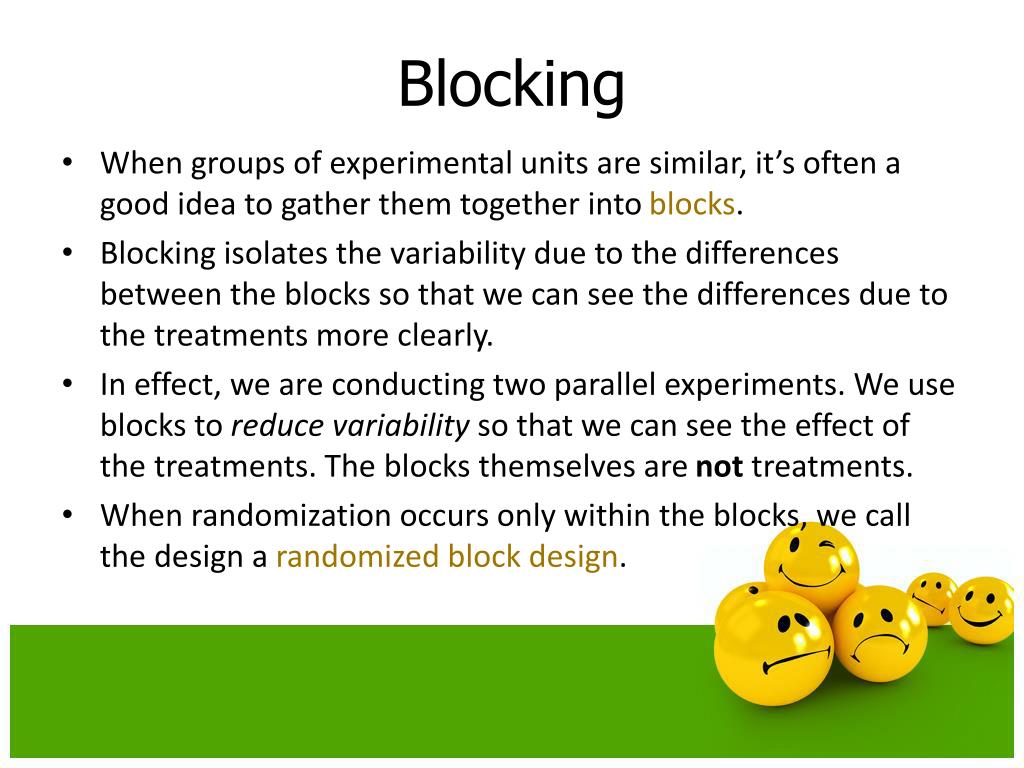
In Design of Experiments, blocking involves recognizing uncontrolled factors in an experiment–for example, gender and age in a medical study–and ensuring as wide a spread as possible across these nuisance factors. Let’s take participant gender in a simple 3-factor experiment as an example. Here is an actual data example for a design balanced for carryover effects. In this example the subjects are cows and the treatments are the diets provided for the cows. Using the two Latin squares we have three diets A, B, and C that are given to 6 different cows during three different time periods of six weeks each, after which the weight of the milk production was measured. We have not randomized these, although you would want to do that, and we do show the third square different from the rest.
Stop blocking postdocs’ paths to success - Nature.com
Stop blocking postdocs’ paths to success.
Posted: Thu, 31 Aug 2017 07:00:00 GMT [source]
Allocate your observations into treatments
Note that Case 2 may also be flipped where you might have the same machines, but different operators. Where F stands for “Full” and R stands for “Reduced.” The numerator and denominator degrees of freedom for the F statistic is \(df_R - df_F\) and \(df_F\) , respectively. Remember, the hardness of specimens (coupons) is tested with 4 different tips. Imagine an extreme scenario where all of the athletes that are running on turf fields get allocated into one group and all of the athletes that are running on grass fields are allocated into the other group.
ANOVA Display for the RCBD
The explanatory variable is the diet pill and the response variable is the amount of weight loss. Although the sex of the patient is not the main focus of the experiment—the effect of the drug is—it is possible that the sex of the individual will affect the amount of weight lost. The remaining four combinations are use in the second block of experiments.
Randomized Block Design (RBD)
The reason for grouping experimental units is so that the observed differences between treatments will be largely due to “true” differences between treatments and not random occurrences or chance. If the number of times treatments occur together within a block is equal across the design for all pairs of treatments then we call this a balanced incomplete block design (BIBD). Here we have treatments 1, 2, up to t and the blocks 1, 2, up to b.
Identify nuisance variables
This type of experimental design is surprisingly powerful and often results in a high probability to create a near optimal design. If you look at how we have coded data here, we have another column called residual treatment. For the first six observations, we have just assigned this a value of 0 because there is no residual treatment. But for the first observation in the second row, we have labeled this with a value of one indicating that this was the treatment prior to the current treatment (treatment A). In this way the data is coded such that this column indicates the treatment given in the prior period for that cow.
What we did here was use the one-way analysis of variance instead of the two-way to illustrate what might have occurred if we had not blocked, if we had ignored the variation due to the different specimens. Ok, with this scenario in mind, let's consider three cases that are relevant and each case requires a different model to analyze. The cases are determined by whether or not the blocking factors are the same or different across the replicated squares. The treatments are going to be the same but the question is whether the levels of the blocking factors remain the same. Therefore, one can test the block simply to confirm that the block factor is effective and explains variation that would otherwise be part of your experimental error. However, you generally cannot make any stronger conclusions from the test on a block factor, because you may not have randomly selected the blocks from any population, nor randomly assigned the levels.
Fragment-based de novo design of a cystathionine γ-lyase selective inhibitor blocking hydrogen sulfide production ... - Nature.com
Fragment-based de novo design of a cystathionine γ-lyase selective inhibitor blocking hydrogen sulfide production ....
Posted: Thu, 06 Oct 2016 07:00:00 GMT [source]
The next thing you need to do after you determine your blocking factors is allocate your observations into blocks. To simplify things, we will assume that you have one main blocking factor that you want to balance over. First the individual observational units are split into blocks of observational units that have similar values for the key variables that you want to balance over. After that, the observational units from each block are evenly allocated into treatment groups in a way such that each treatment group is allocated similar numbers of observational units from each block. With the increasing maturity of the text-to-image and image-to-image generative models, AI-generated images (AGIs) have shown great application potential in advertisement, entertainment, education, social media, etc.
In addition, considering the correlation between the image and prompt, AMFF-Net compares the semantic features from text encoder and image encoder to evaluate the text-to-image alignment. We carry out extensive experiments on three AGI quality assessment databases, and the experimental results show that our AMFF-Net obtains better performance than nine state-of-the-art blind IQA methods. The results of ablation experiments further demonstrate the effectiveness of the proposed multi-scale input strategy and AFF block. The ideal experimental design is the randomized controlled double-blind experiment. Since the first three columns contain some pairs more than once, let's try columns 1, 2, and now we need a third...how about the fourth column. If you look at all possible combinations in each row, each treatment pair occurs only one time.
Week one would be replication one, week two would be replication two and week three would be replication three. Variability between blocks can be large, since we will remove this source of variability, whereas variability within a block should be relatively small. In general, a block is a specific level of the nuisance factor.
In our previous diet pills example, a blocking factor could be the sex of a patient. We could put individuals into one of two blocks (male or female). And within each of the two blocks, we can randomly assign the patients to either the diet pill (treatment) or placebo pill (control).
For instance, if you had a plot of land the fertility of this land might change in both directions, North -- South and East -- West due to soil or moisture gradients. As we shall see, Latin squares can be used as much as the RCBD in industrial experimentation as well as other experiments. If the structure were a completely randomized experiment (CRD) that we discussed in lesson 3, we would assign the tips to a random piece of metal for each test.
In this case it would be near impossible to separate the impact that the type of cleats has on the run times from the impact that the type of field has.
No comments:
Post a Comment